Isaac ROS Depth Segmentation
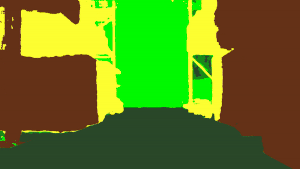
Overview
Isaac ROS Depth Segmentation provides NVIDIA NVIDIA-accelerated packages for
depth segmentation. The isaac_ros_bi3d
package uses the
optimized Bi3D DNN
model
to perform stereo-depth estimation via binary classification, which is
used for depth segmentation. Depth segmentation can be used to
determine whether an obstacle is within a proximity field and to avoid
collisions with obstacles during navigation.
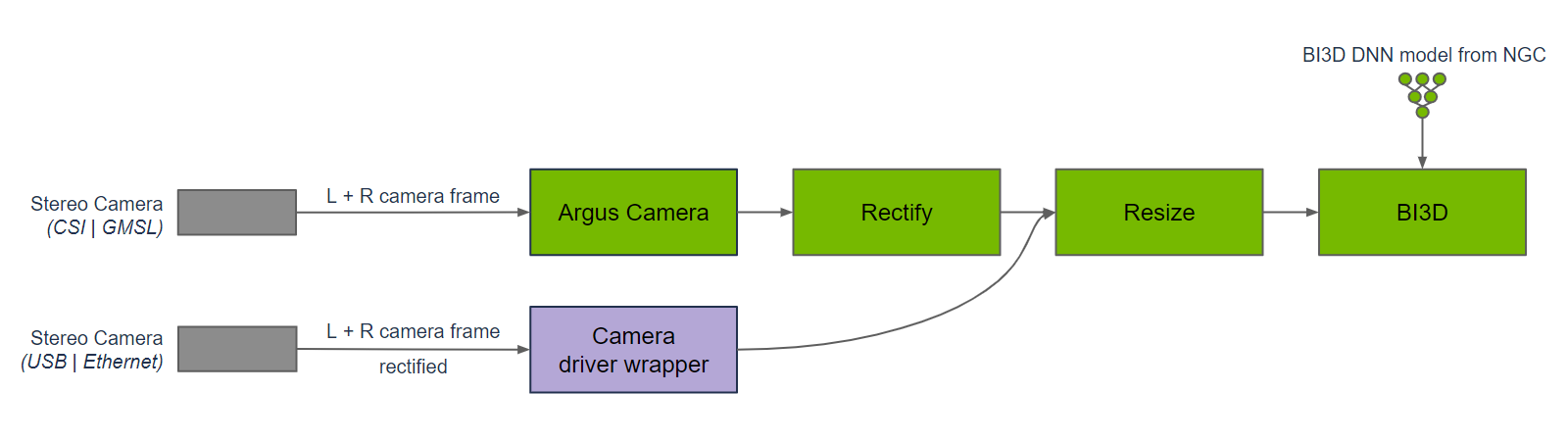
Bi3D is used in a graph of nodes to provide depth segmentation from a time-synchronized input left and right stereo image pair. Images to Bi3D need to be rectified and resized to the appropriate input resolution. The aspect ratio of the image needs to be maintained; hence, a crop and resize may be required to maintain the input aspect ratio. The graph for DNN encode, to DNN inference, to DNN decode is part of the Bi3D node. Inference is performed using TensorRT, as the Bi3D DNN model is designed to use optimizations supported by TensorRT.
Compared to other stereo disparity functions, depth segmentation provides a prediction of whether an obstacle is within a proximity field, as opposed to continuous depth, while simultaneously predicting freespace from the ground plane, which other functions typically do not provide. Also unlike other stereo disparity functions in Isaac ROS, depth segmentation runs on NVIDIA DLA (deep learning accelerator), which is separate and independent from the GPU. For more information on disparity, refer to this page.
Note
This DNN is optimized for and evaluated with RGB global shutter camera images, and accuracy may vary on monochrome images.
Quickstarts
Isaac ROS NITROS Acceleration
This package is powered by NVIDIA Isaac Transport for ROS (NITROS), which leverages type adaptation and negotiation to optimize message formats and dramatically accelerate communication between participating nodes.
Packages
Supported Platforms
This package is designed and tested to be compatible with ROS 2 Humble running on Jetson or an x86_64 system with an NVIDIA GPU.
Note
Versions of ROS 2 other than Humble are not supported. This package depends on specific ROS 2 implementation features that were introduced beginning with the Humble release. ROS 2 versions after Humble have not yet been tested.
Platform |
Hardware |
Software |
Notes |
---|---|---|---|
Jetson |
For best performance, ensure that power settings are configured appropriately. Jetson Orin Nano 4GB may not have enough memory to run many of the Isaac ROS packages and is not recommended. |
||
x86_64 |
|
Docker
To simplify development, we strongly recommend leveraging the Isaac ROS Dev Docker images by following these steps. This will streamline your development environment setup with the correct versions of dependencies on both Jetson and x86_64 platforms.
Note
All Isaac ROS Quickstarts, tutorials, and examples have been designed with the Isaac ROS Docker images as a prerequisite.
Customize your Dev Environment
To customize your development environment, reference this guide.
Updates
Date |
Changes |
---|---|
2024-09-26 |
Update for ZED compatibility |
2024-05-30 |
Update to be compatible with JetPack 6.0 |
2023-10-18 |
Renamed repository to |
2023-05-25 |
Performance improvements |
2023-04-05 |
Source available GXF extensions |
2022-08-31 |
Update to use latest model and to be compatible with JetPack 5.0.2 |
2022-06-30 |
Initial release |